An Overview of Instruction Tuning Data
This post covers a range of widely used instruction tuning datasets, as well as important characteristics of instruction tuning data and best practices for using the datasets.
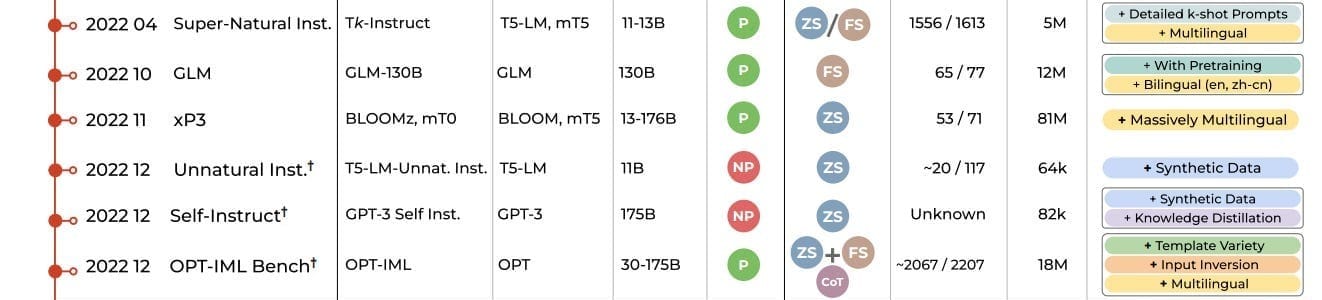
NLP and ML have gone through several phases of how models are trained in recent years. With the arrival of pre-trained models such as BERT, fine-tuning pre-trained models for downstream tasks became the norm. The increasing capabilities of ever larger models then enabled in-context learning via prompting. Recently, instruction tuning has become the newest method to make LLMs useful.
This post covers a range of widely used instruction tuning datasets, as well as important characteristics of instruction tuning data and best practices for using the datasets.
This post was first published in NLP News in two articles.
What is Instruction Tuning?
The main difference between instruction tuning and standard supervised fine-tuning lies in the data that the model is trained on. Whereas supervised fine-tuning trains models on input examples and their corresponding outputs, instruction tuning augments input-output examples with instructions, which enables instruction-tuned models to generalize more easily to new tasks.
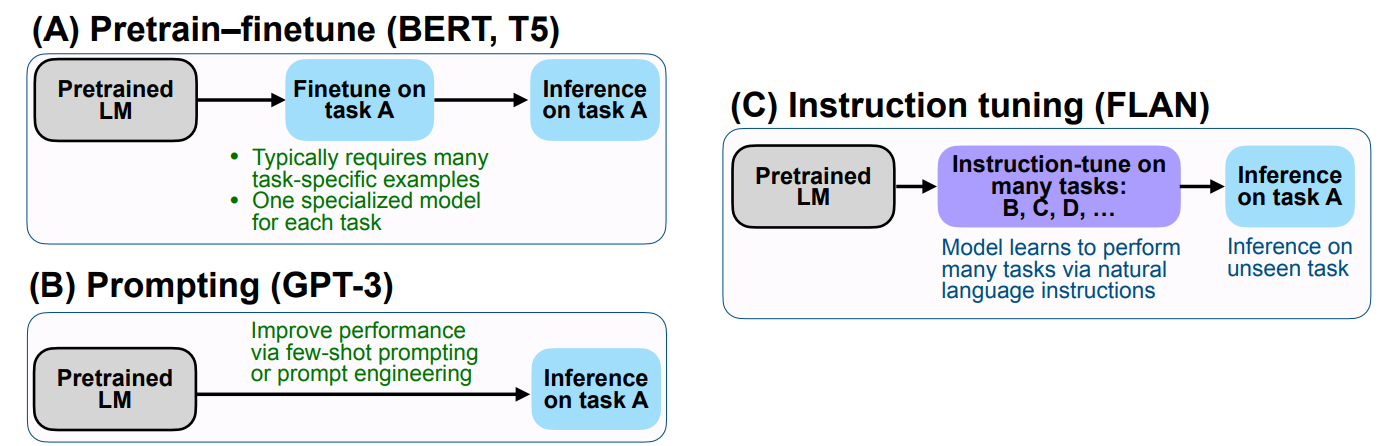
Methods differ based on how the instruction tuning data is constructed. Zhang et al. (2023) provide a good overview of existing instruction datasets. Existing datasets fall roughly into two main categories: a) instructions are added to existing NLP tasks; and b) data from (a) is used to condition a model to generate new instruction-input-output tuples.
Important Data Characteristics
There are a several aspects to consider when using instruction tuning datasets:
- Data source: How was the data obtained? Most datasets have been generated using ChatGPT. They may thus inherit biases of the source model or may be noisy. Human-written examples are more expensive to obtain but are more high quality.
- Data quality: Was any filtering done to improve the quality of the generated data? In most cases, filtering is based on simple heuristics or a pre-trained model, which can result in noisy data. For instance, the authors of OpenAssistant Conversations went the extra mile and obtained human-annotated data quality labels.
- Domain and language coverage: Most datasets cover general QA-style use cases and are in English. However, similar methods can be used to obtain data in other domains or languages.
- Number of dialog turns: A dialog turn is an utterance by one speaker. Most datasets are single-turn, i.e., they consist of a prompt and a single response. Multi-turn data may be necessary to train a more conversational model.
- License terms: Data generated using OpenAI models is subject to the OpenAI terms of use, which prohibit using the data to develop competing models. So look for data with a more permissive license to avoid any legal complications.
Instruction Tuning Datasets
Let us now look at a representative set of widely used instruction tuning datasets, in order of their release:
Natural Instructions (Mishra et al., 2022): 193k instruction-output examples sourced from 61 existing English NLP tasks. Crowd-sourcing instructions from each dataset are aligned to a common schema. Instructions are thus more structured compared to other datasets. Outputs are relatively short, however, which makes the data less useful for generating long-form content.
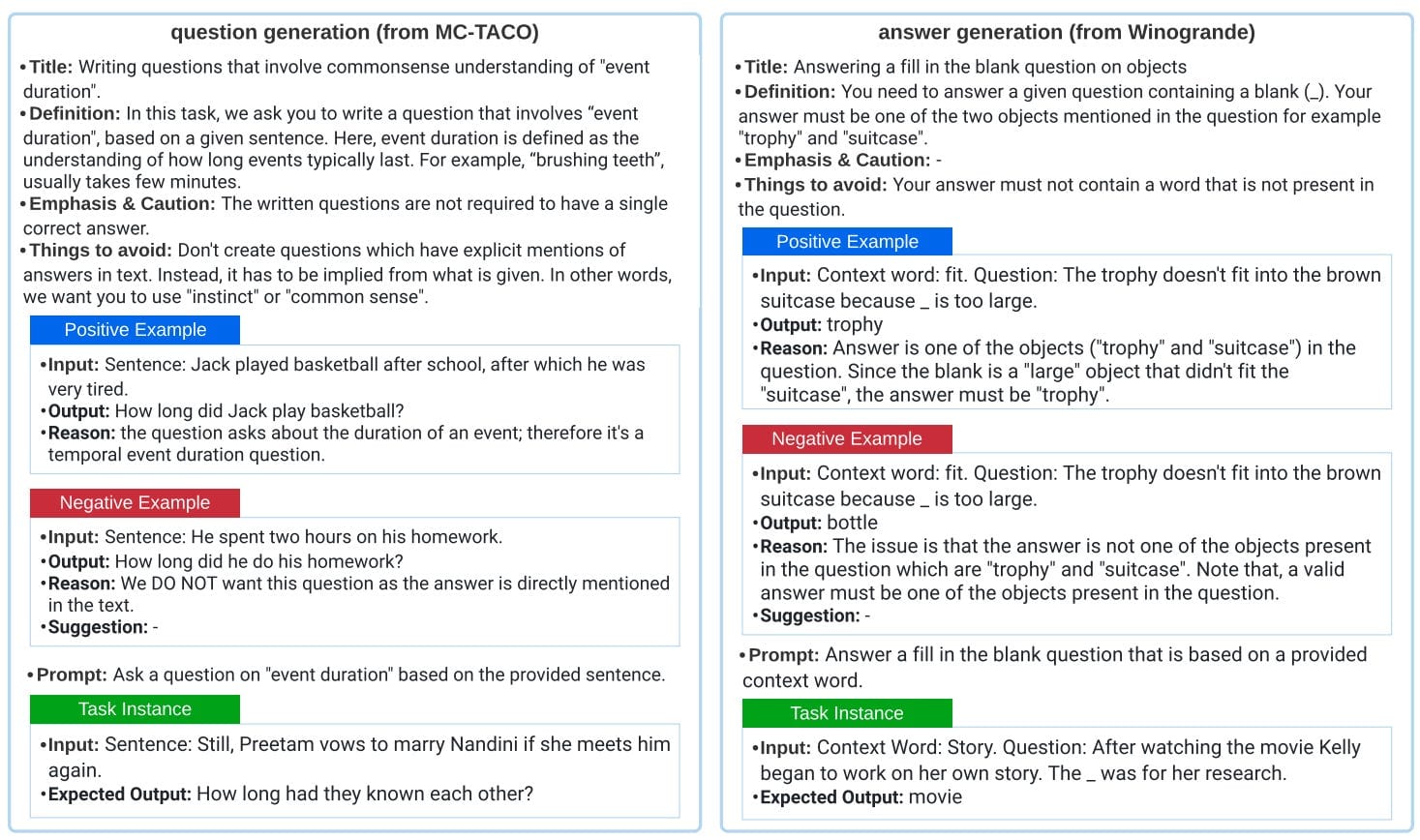
Natural Instructions v2 / Super-Natural Instructions (Wang et al., 2022): A crowd-sourced collection of instruction data based on existing NLP tasks and simple synthetic tasks. It includes 5M examples across 76 tasks in 55 languages. Compared to Natural Instructions, instructions are simplified; they consist of a task definition and positive and negative examples with explanations.
Unnatural Instructions (Honovich et al., 2023): An automatically collected instruction dataset of 240k examples where InstructGPT (text-davinci-002) is prompted with three Super-Natural Instructions examples—consisting of an instruction, input, possible output constraints—and asked to generate a new example. The output is generated separately by conditioning on the generated instruction, input, and constraints. The generated instructions are then further paraphrased by prompting the model. Unnatural Instructions covers a more diverse set of tasks than Super-Natural Instructions; while many examples reflect classical NLP tasks, it also includes examples of other interesting tasks.
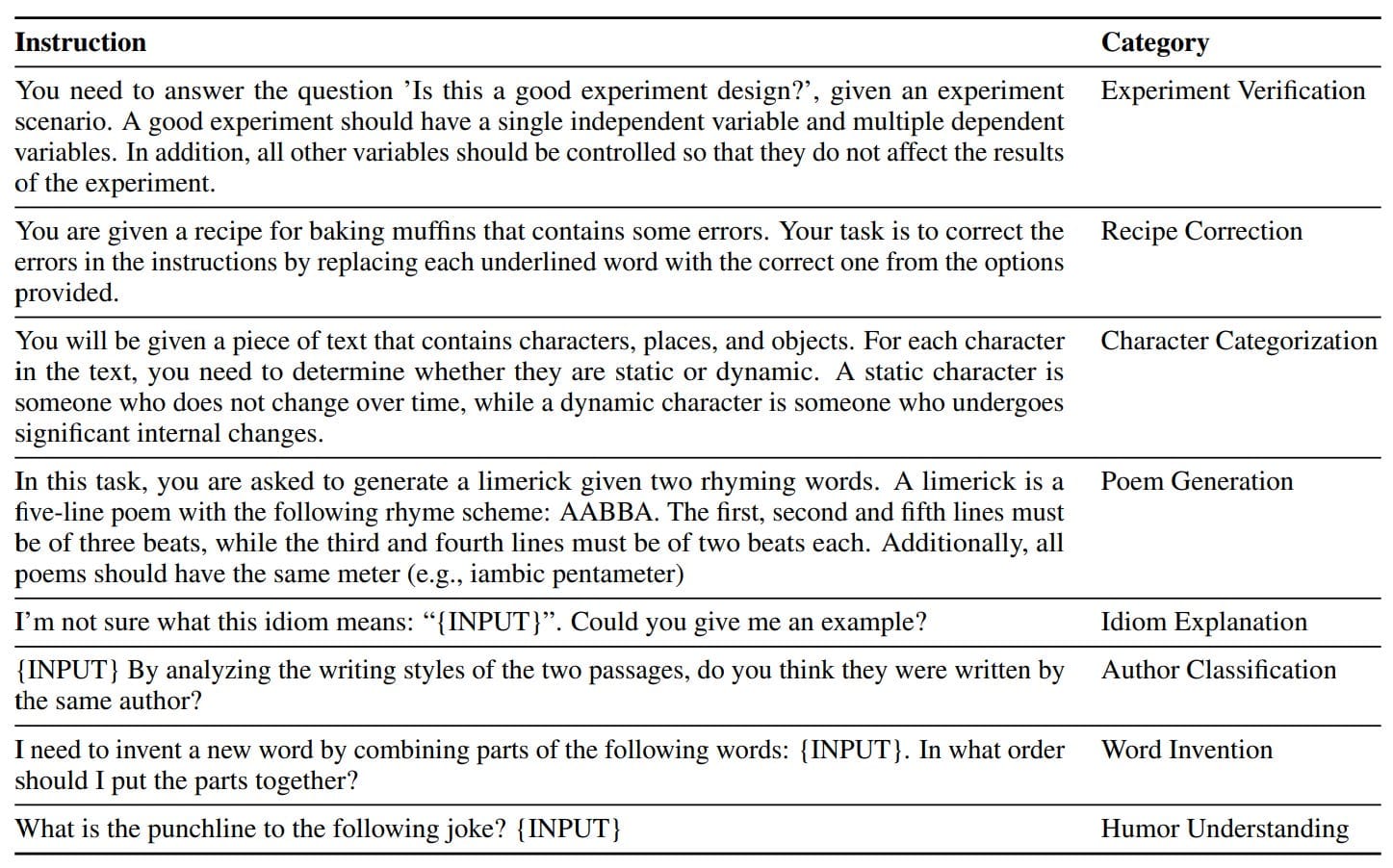
Self-Instruct (Wang et al., 2023): Similar to Unnatural Instructions, Self-Instruct consists of 82k examples automatically generated using InstructGPT conditioned on a set of seed task examples (175 tasks in total; one example per task; 8 examples are sampled for in-context learning). Self-Instruct decouples the example generation by first generating the instruction, then the input (conditioned on instruction), and then the output. For classification tasks, the authors first generate the possible output labels and then condition the input generation on each class label to avoid biasing towards a specific label. While the generated instructions are mostly valid, the generated outputs are often noisy.
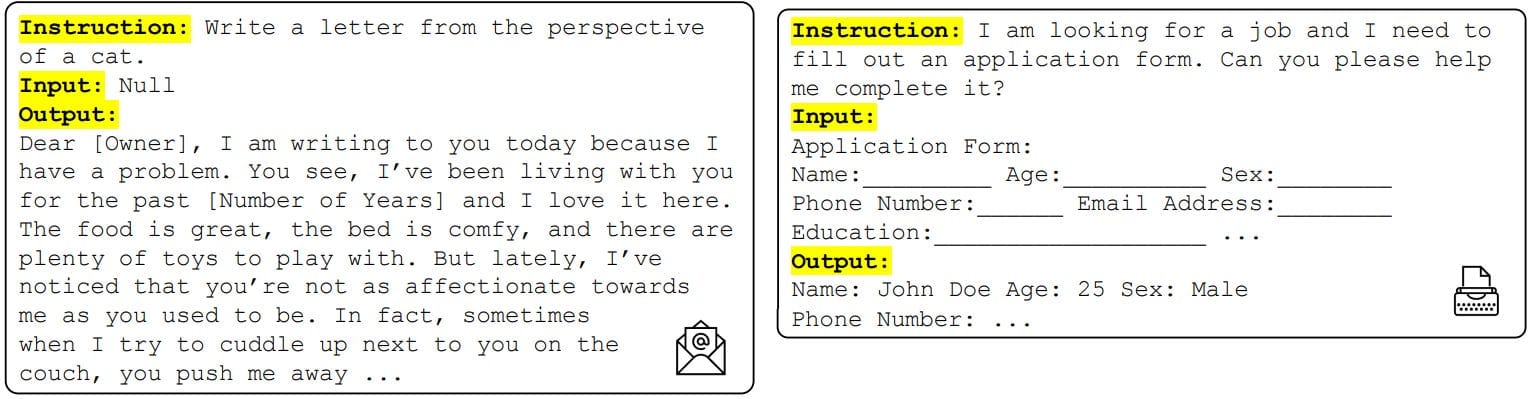
P3 (Public Pool of Prompts; Sanh et al., 2022): A crowd-sourced collection of prompts for 177 English NLP tasks. For each dataset, about 11 different prompts are available on average, which enables studying the impact of different prompt formulations. Compared to the instructions in the above instruction datasets, P3 prompts are often shorter and less elaborate.
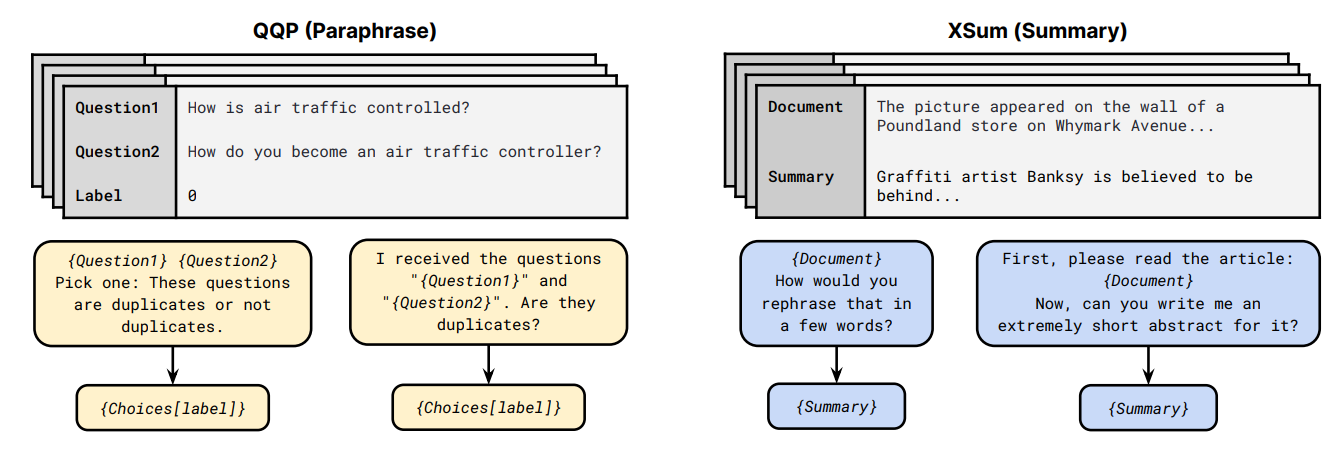
xP3, xP3mt (Muennighoff et al., 2023): An extension of P3 including 19 multilingual datasets and 11 code datasets, with English prompts. They also release a machine-translated version of the data (xP3mt), which contains prompts automatically translated into 20 languages. Fine-tuning on multilingual tasks with English prompts further improves performance beyond only fine-tuning on English instruction data.
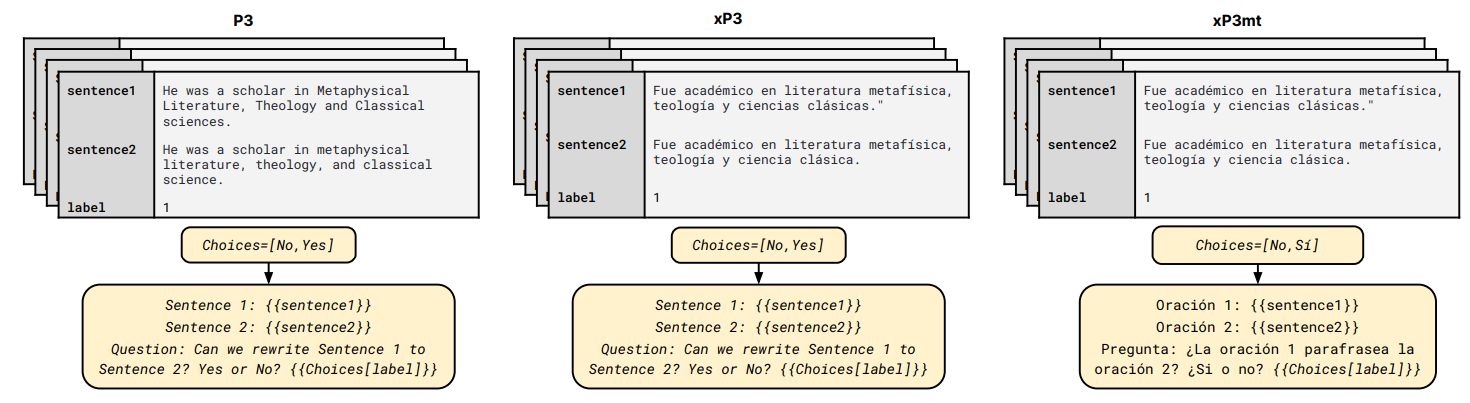
Flan 2021 / Muffin (Wei et al., 2022): Prompts for 62 English text datasets, with 10 prompt templates for each task. For classification tasks, an OPTIONS
suffix is appended to the input in order to indicate output constraints.
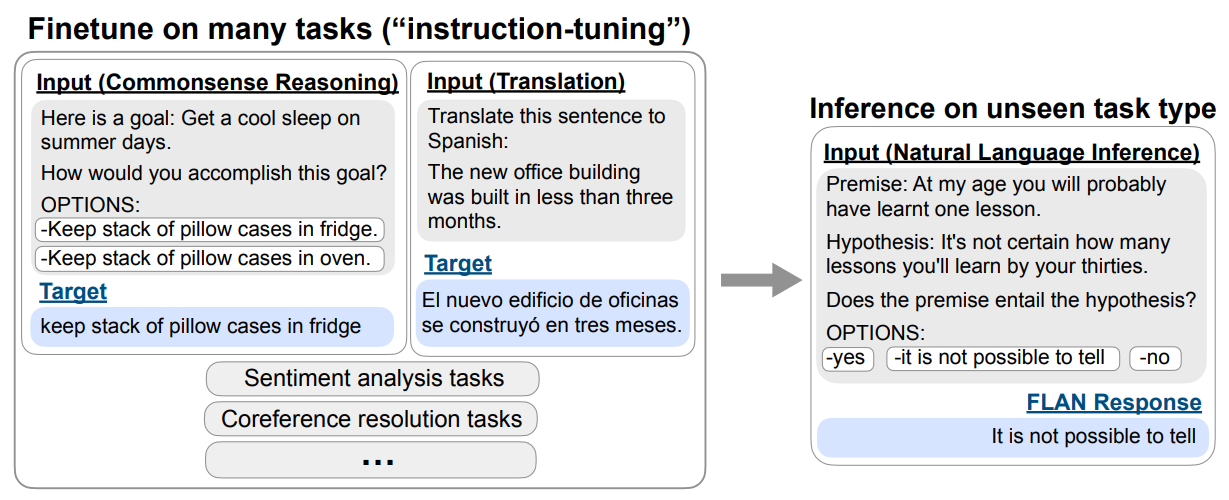
Flan 2022 (Chung et al., 2022): A combination of Flan 2021, P3, Super-Natural Instructions, and additional reasoning, dialog, and program synthesis datasets. The 9 new reasoning datasets are additionally annotated with chain-of-thought (CoT; Wei et al., 2022) annotations.
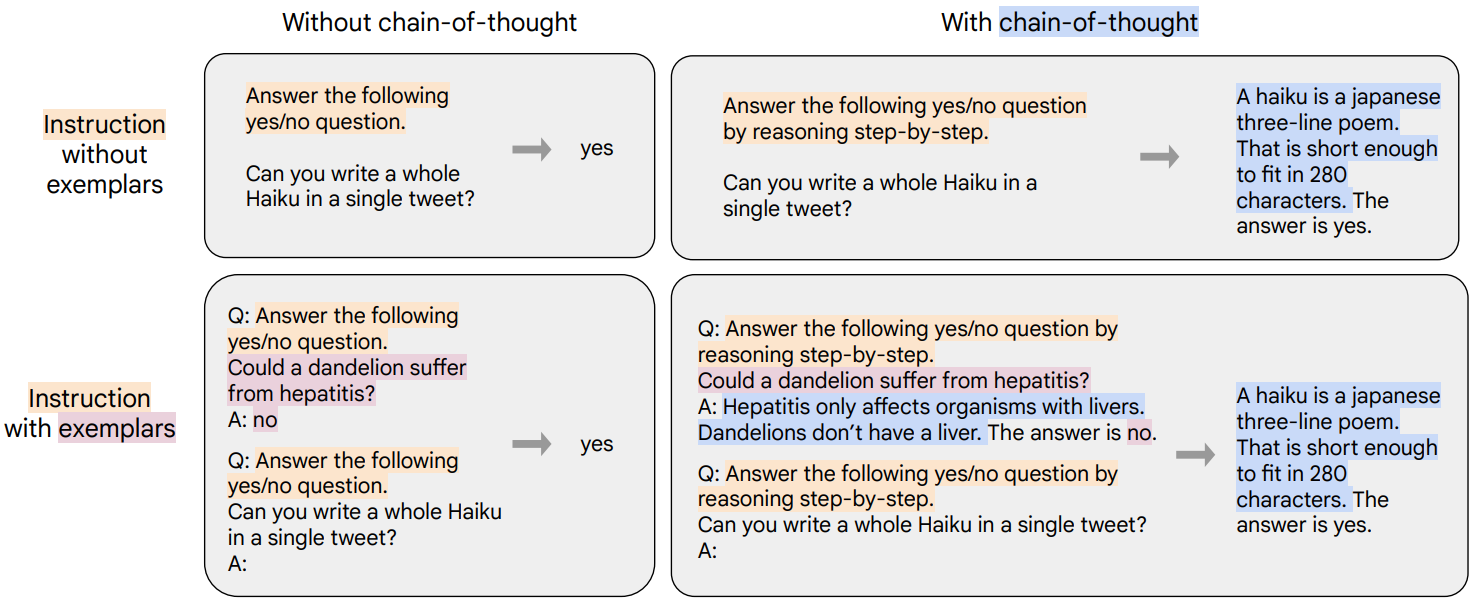
Opt-IML Bench (Iyer et al., 2022): A combination of Super-Natural Instructions, P3, and Flan 2021. They additionally include dataset collections on cross-task transfer, knowledge grounding, dialogue, and a larger number of chain-of-thought datasets.
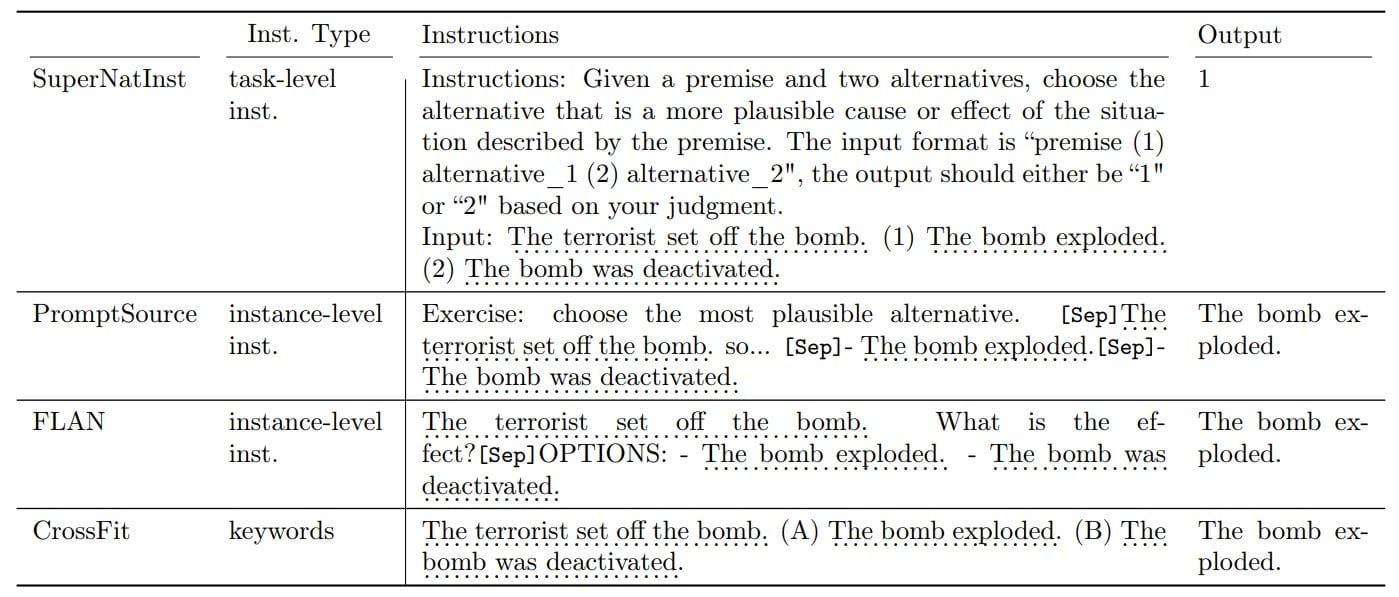
Alpaca data (Taori et al., March 2023): 52k English instruction examples generated using OpenAI’s text-davinci-003 with self-instruct. The authors applied some modifications to simplify the data generation pipeline and lower costs—the final data cost less than $500 to generate!
Evol-Instruct (Xu et al., April 2023): A rewritten set of 250k English instruction-response pairs based on the Alpaca data. Instructions are rewritten a) to make them more complex or b) to create a new, more specialized instruction by prompting ChatGPT. In a second step, ChatGPT is used to generate the corresponding responses. Low-quality instruction-response pairs are filtered using heuristics. This process is repeated three times.
Vicuna ShareGPT data (Chiang et al., March 2023): 70k English conversations shared by users and scraped from sharegpt.com. Pre-processing involved converting HTML to markdown, filtering out low-quality samples, and splitting lengthy conversations into smaller segments. Compared to the above single-turn datasets, the ShareGPT conversations often consist of multiple turns and are thus more useful for training a model to leverage the context of the conversation. The conversations may be owned by the users so their use is potentially problematic.
Baize data (Xu et al., April 2023): 54k and 57k English multi-turn dialog examples (3.4 turns on average) generated with ChatGPT using questions from Quora and StackOverflow datasets respectively as seeds. ChatGPT simulates both the human and AI participants of the conversation.1 In addition, they also generated 47k dialogs in the medical domain based on MedQuAD questions.
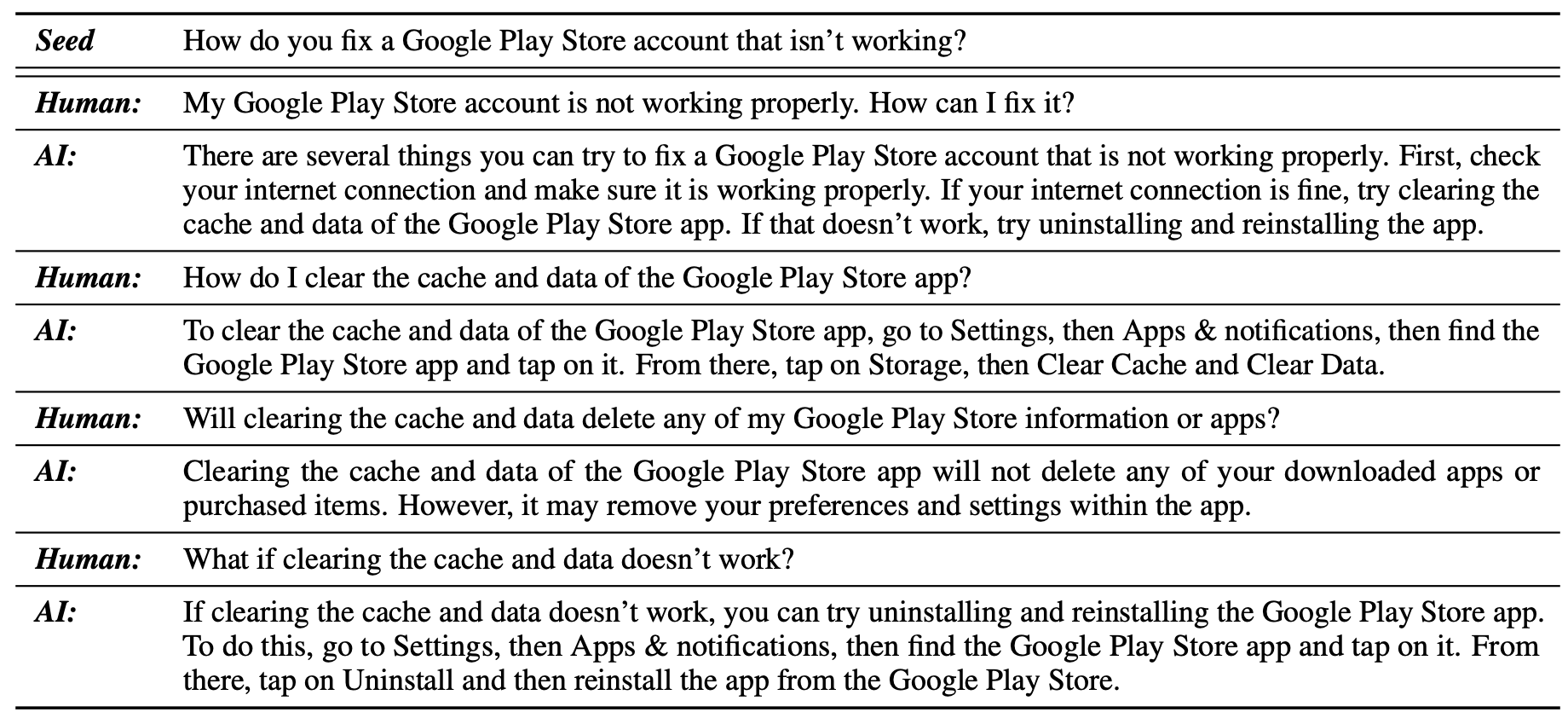
databricks-dolly-15k (Conover et al., April 2023): 15k English instruction-following examples written by Databricks employees. Crucially, both instructions and answers are human-generated. This in contrast to the other datasets above where instruction and/or answers are generated by ChatGPT. Examples cover 7 use cases: open QA, closed QA, information extraction and summarization of Wikipedia data, brainstorming, classification, and creative writing. Compared to the other datasets above, the data is released under a permissive license that also allows for commercial use.
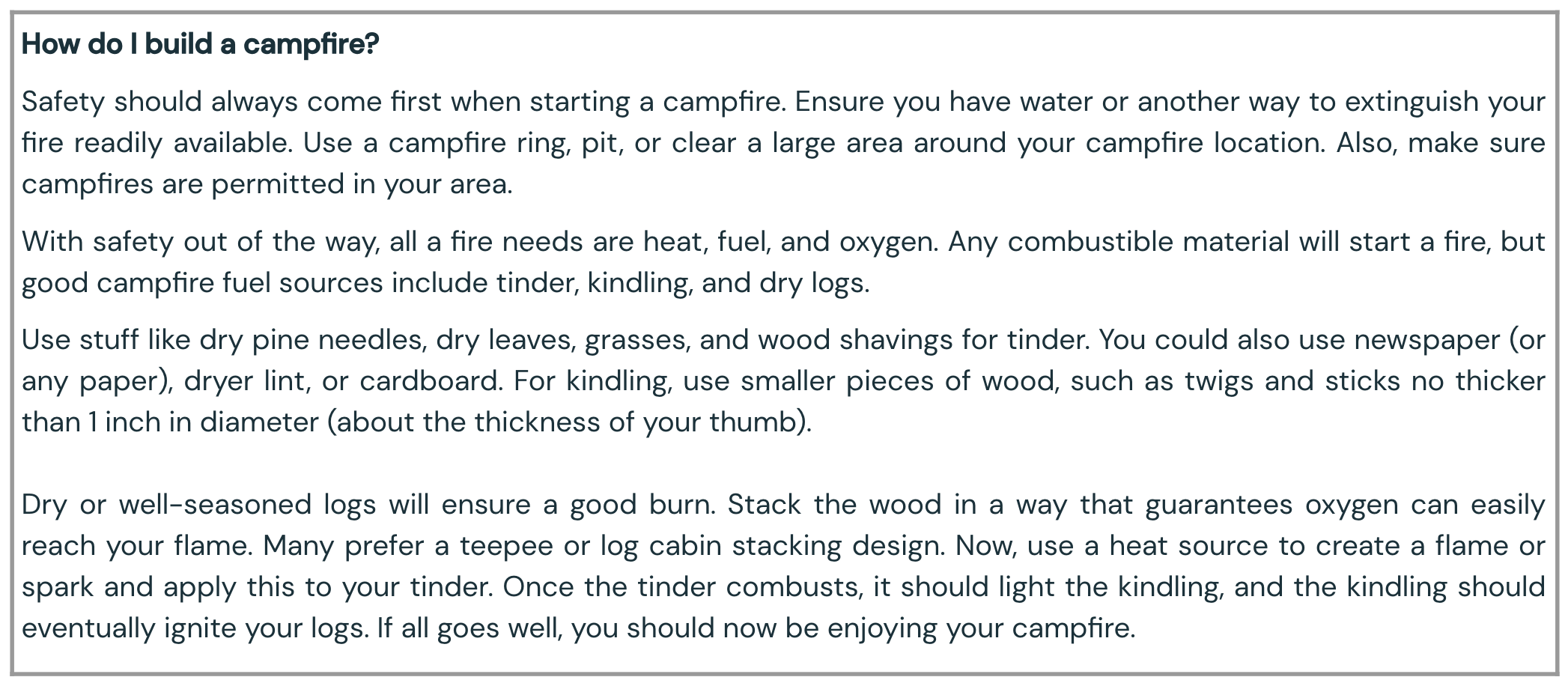
databricks-dolly-15k
.OpenAssistant Conversations (Köpf et al., April 2023): 11k crowd-sourced multilingual instruction-following conversations (for 52k examples, only the prompts are available). Human annotators generated messages for both the assistant and the human participant. The data differs in several aspects from the other datasets: 1) it is multilingual (42.8% examples are in English, 31.4% in Spanish, and the rest in other languages); 2) annotators annotated the quality of prompts and responses (460k quality ratings overall); and 3) the annotators were provided with detailed guidelines, both for writing prompts and for acting as the assistant. The data uses a permissive license, which allows commercial use.
LIMA data (Zhou et al., May 2023): 1k training and 300 test answer–response pairs mostly sampled from StackExchange, wikiHow and the Pushshift Reddit dataset with around 400 written by the paper authors. A nice observation of this study is that training on this small set of curated instruction data outperforms training on the much larger, noisier Alpaca data.
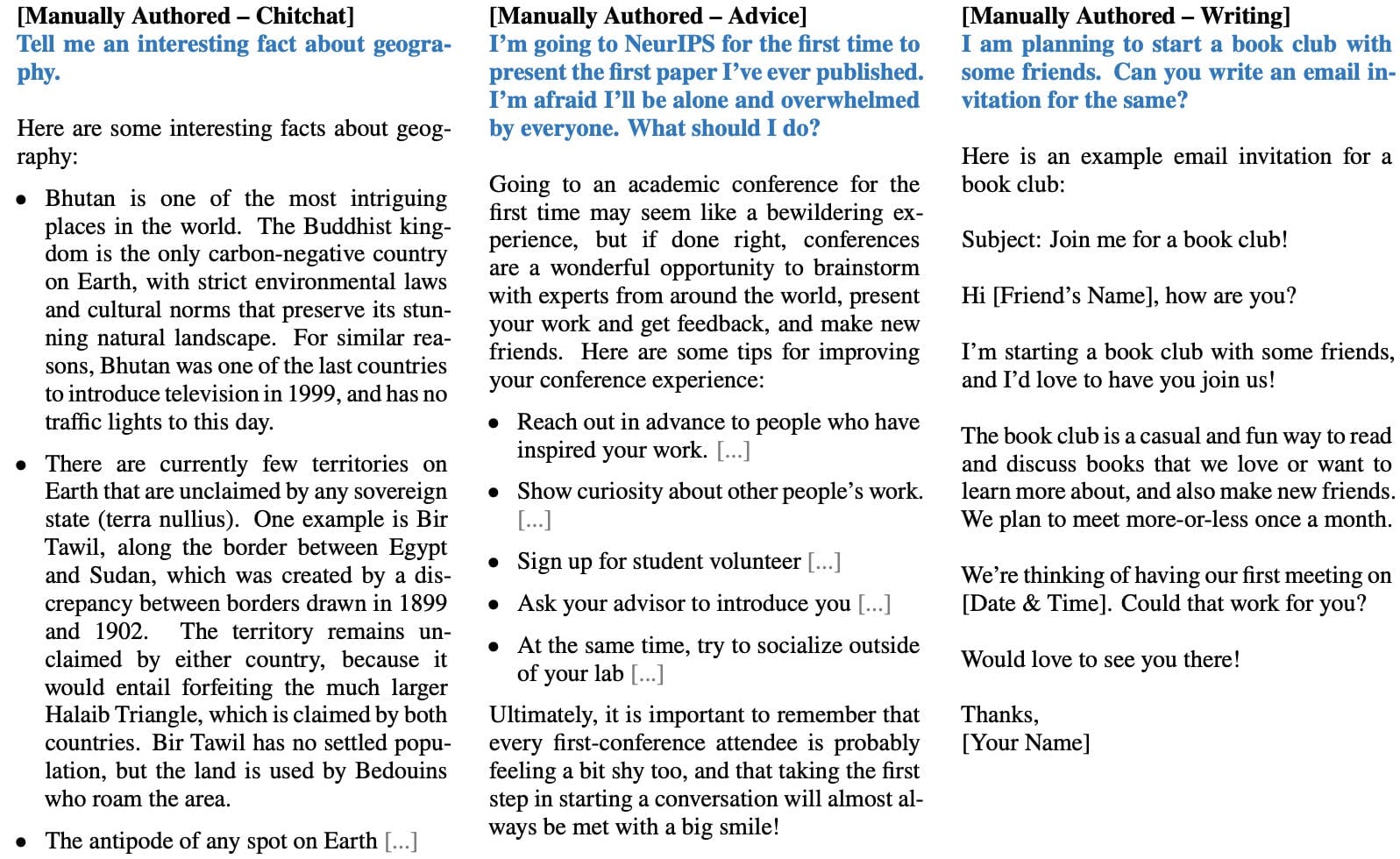
Instruction Tuning Best Practices
Longpre et al. (2023) and Iyer et al. (2022) ablate several important aspects of using instruction tuning data:
Mixing few-shot settings. Training with mixed zero-shot and few-shot prompts significantly improves performance in both settings.
Task diversity. Large models benefit from continuously increasing the number of tasks.
Data augmentation. Augmenting the data such as by inverting inputs and outputs (e.g., turning a question answering task into a question generation task) is beneficial.
Mixing weights. When using a combination of instruction tuning datasets, appropriately tuning the mixing weights is important.
Takeaways
✅ Quality > quantity. As Zhou et al. (2023) observe, training on a small set of high-quality data outperforms instruction-tuning on larger, noisier data. Using more diverse prompts and quality filtering both improve performance.
🧑🎓 Imitation != mastery. Models that are instruction-tuned on ChatGPT-generated data mimic ChatGPT’s style (and may thus fool human raters!) but not its factuality (Gudibande et al., May 2023). They perform worse on standard benchmarks. Using stronger base models is the best way to address this.
🏛️ The stronger the base, the better. More powerful base models also produce stronger instruction-tuned models (Wang et al., June 2023).
🥇 Combination wins. Combining multiple instruction-tuning datasets results in the best average performance across tasks (Wang et al., June 2023). Dataset mixing and developing modular instruction-tuned models are thus important research directions.
Future Directions
Understanding instruction-tuning. While we have seen a proliferation of instruction-tuning datasets, we still lack a clear understanding of what makes a good instruction and good instruction–response pairs. There is much anecdotal knowledge when it comes to creating good model prompts—but to my knowledge it is unclear how instruction–following data can be created at scale in a more principled manner.
Improving data quality. To improve model performance, we need to develop more reliable methods to identify high-quality examples and filter out undesirable ones. In a similar vein, it is important to develop methods that allow us to identify how a particular instance affects model behavior and alignment at test time.
Evaluating instruction-tuned models. In light of the biases of both human and automatic evaluations, there is no clear gold standard for how to evaluate instruction-tuned models. Evaluating a model on a set of tests that can be efficiently and automatically evaluated is one way to side-step this issue, see LMentry (Efrat et al., ACL 2023), M2C (Hlavnova & Ruder, ACL 2023), IFEval (Zhou et al., Nov 2023), etc but these are restricted to a certain set of use cases. In general, it is crucial to design evaluations with a target application in mind.